1st Workshop on
MACHINE LEARNING
UNDER
WEAKLY STRUCTURED INFORMATION
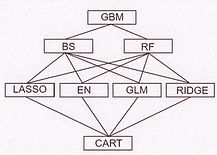
_edited.png)
Each talk will consist of (approximately) 20 minutes presentation plus 15 minutes Q&A seesion. Additionally, there will be a discussion session on Friday before dinner. In this session, participants will be given the opportunity to have informal chats on specific talks (and beyond).
​
​
Schedule:
​
Day I (03.05.2024)
12:30 - 12:55: Opening (Christoph Jansen and Hannah Blocher)
​
13:00 - 13:35: Talk 1 (Thomas Augustin)
Title: 50+ Years of Uncertainty Quantification @Stat.LMU: Some Partially Historical Remarks
Abstract: The talk opens the workshop with partially historical remarks on the Department of Statistics and (!) Philosophy of Science at LMU Munich. During its founding decades, research activities focused on questions concerning the foundations of statistics. First, we quickly trace back Weichselberger's (and Stegmüller's) search for a general inference theory and then review its impact on knowledge representation in expert systems and credal models in machine learning. Secondly, we recall Schneeweiß's work on measurement error modelling in regression models and then discuss the relevance of some of those questions and techniques for predictive modelling, particularly interpretable machine learning.
​
13:40 - 14:15: Talk 2 (Christian Fröhlich)
Title: Scoring Rules and Calibration for Imprecise Probabilities
Abstract: The theory of evaluating precise probabilistic forecasts is well-established, and is based on the key concepts of proper scoring rules of calibration. Our goal is to find evaluation criteria for imprecise forecasts and to address when such imprecise forecasts are warranted. For this reason, we introduce generalizations of proper scoring rules and calibration for imprecise probabilities.
​
14:20 - 14:55: Talk 3 (Yusuf Sale)
Title: Label-wise Aleatoric and Epistemic Uncertainty Quantification
Abstract: We present a novel approach to uncertainty quantification in classification tasks based on label-wise decomposition of uncertainty measures. This label-wise perspective allows uncertainty to be quantified at the individual class level, thereby improving cost-sensitive decision-making and helping understand the sources of uncertainty. Furthermore, it allows to define total, aleatoric and epistemic uncertainty on the basis of non-categorical measures such as variance, going beyond common entropy-based measures. In particular, variance-based measures address some of the limitations associated with established methods that have recently been discussed in the literature. We show that our proposed measures adhere to a number of desirable properties. Through empirical evaluation on a variety of benchmark data sets---including applications in the medical domain where accurate uncertainty quantification is crucial---we establish the effectiveness of label-wise uncertainty quantification.
​
15:00 - 15:35: Talk 4 (Lisa Wimmer)
Title: Causal misspecification in Bayesian inference
Abstract: We study the implications of causal relations for the estimation of (predictive) uncertainty in machine learning (ML) tasks. Our approach challenges the ML paradigm of refraining from making assumptions about the data-generating process and betting everything on the observed data instead. While this tends to work well in terms of predictive performance, several scholars have noted undesirable phenomena, with models fooled into detecting spurious relations, that can be attributed to the total disregard of causal relationships. We conjecture that the consequences of anti-causal modeling on uncertainty quantification (UQ) might be similarly detrimental.
​
—------------------------ Coffee Break —-------------------------------------------
16:10 - 16:45: Talk 5 (Tom Sterkenburg)
Title: Universal prediction
Abstract: I will give a critical discussion of the Solomonoff-Levin theory of universal sequential prediction, which is built on Kolmogorov complexity.
Historically, the work of Ray Solomonoff was inspired by a research program in the philosophy of science that aimed to develop a formal logic of inductive inference. Rudolf Carnap started working on his "inductive logic" in the 1940's, and, together with many collaborators (including, in Munich, Wolfgang Stegmüller) continued adapting and refining this logic for the last decades of his life. However, already in the 1950's, Hilary Putnam gave a diagonalization proof that he took to be the nail in the coffin for Carnap's program. I will discuss how (a generalization of) Putnam's diagonalization argument also spells trouble for the claim that the Solomonoff-Levin theory delivers a universal prediction method.
Finally, time permitting, I will comment on some more recent appearances of the Solomonoff-Levin theory in attempts to account for the generalization success of deep learning.
​
16:50 - 17:25: Talk 6 (Georg Schollmeyer)
Title: A Short Note on Idempotent Learners, Empirical Risk Minimization and the Necessary Condition in VC Theory
Abstract: Many classical regression-, estimation- and classification approaches both from statistics and from machine learning have an intuitively appealing idempotency property. This is itself due to the often followed conceptual approach of projecting observed noisy data onto a space of parameters/functions, or more correctly, to their corresponding predictions. As a projection is particularly idempotent, these methods take this property over. Examples range from linear or polynomial regression to methods of empirical risk minimization like support vector machines.
On the other hand, there are also many methods that are not idempotent, for example kernel density estimation, or nearest neighbor approaches. To my personal knowledge, a systematic disambiguation between idempotent and non-idempotent methods within textbooks both in machine learning as well as in statistics is seldomly emphasized. This may be a little bit surprising: Especially in machine learning, empirical risk minimization is often presented as a basic principle whereas other approaches are presented somehow isolatedly without relating them to empirical risk minimization. In contrast, it can be shown that e.g. classification methods can be clearly divided into the class of empirical risk minimizers and the complement of this class, exactly with the dichotomy of idempotent vs non-idempotent classifiers. In this talk I will try to elaborate a little bit on this dichotomy, hoping to shed some light on some conceptual aspects, including (if time allows) the consequences and non-consequences for the necessary condition for uniform convergence in the context of (seemingly) empirical risk minimization.
​
17:30 - 18:05: Talk 7 (Cornelia Gruber)
Title: Sources of Uncertainty in Machine Learning - A Statisticians' View
Abstract: Machine Learning and Deep Learning have achieved an impressive standard today, enabling us to answer questions that were inconceivable a few years ago. Besides these successes, it becomes clear, that beyond pure prediction, which is the primary strength of most supervised machine learning algorithms, the quantification of uncertainty is relevant and necessary as well. While first concepts and ideas in this direction have emerged in recent years, this paper adopts a conceptual perspective and examines possible sources of uncertainty. By adopting the viewpoint of a statistician, we discuss the concepts of aleatoric and epistemic uncertainty, which are more commonly associated with machine learning. The paper aims to formalize the two types of uncertainty and demonstrates that sources of uncertainty are miscellaneous and can not always be decomposed into aleatoric and epistemic. Drawing parallels between statistical concepts and uncertainty in machine learning, we also demonstrate the role of data and their influence on uncertainty.
​
18:10 - 19:15: D&D (Drinks and Discussion)
19:30: Dinner at Georgenhof (dutch treat basis)
Day II (04.05.2024)
​
10:00 - 10:35: Talk 8 (Eyke Hüllermeier)
Title: A Critique of Epistemic Uncertainty Quantification through Second-Order Loss Minimisation
Abstract: Trustworthy machine learning systems should not only return accurate predictions, but also a reliable representation of their uncertainty. Bayesian methods are commonly used to quantify both aleatoric and epistemic uncertainty, but alternative approaches have become popular in recent years, notably so-called evidential deep learning methods. In essence, the latter extend empirical risk minimisation for predicting second-order probability distributions over outcomes, from which measures of epistemic (and aleatoric) uncertainty can be extracted. This presentation will highlight theoretical flaws of evidential deep learning that are rooted in properties of the underlying second-order loss minimisation problem. Starting from a systematic setup that covers a wide range of approaches for classification, regression, and counts, issues regarding identifiability and convergence in second-order loss minimisation will be addressed. Problems regarding the interpretation of the resulting epistemic uncertainty measures and the relative (rather than absolute) nature of such measures will also be discussed.
​
10:40 - 11:15: Talk 9 (Rabanus Derr)
Title: Interpolating between the Good and the Evil - Calibration Error Rates in Sequential Predictions with Stationary to Adversarial Nature
Abstract: Calibration as useful prediction criterion regained traction in the last years. In particular, scholarship elaborated on calibrated predictions in sequential setups. Most work in this realm focused on a fully adversarial nature, i.e. nature draws a distribution from the fully ambiguous interval $[0,1]$ in an adversarial manner and samples from this distribution. It has been shown that in this setting the best achievable calibration error rate is lower bounded by $\Omega(T^{0.528})$. Current prediction algorithms, however, only guarantee $O(T^{2/3})$ vanishing calibration error. If we assume, however, that nature is not fully adversarial but restricted to sample from a stationary, precise ground truth, we provide a simple algorithm which guarantees optimal vanishing calibration error in $O(\sqrt{T})$. Now interpolating between a stationary, precise nature and a fully adversarial nature, we find that the prediction task for any stationary, imprecise interval is as difficult, up to constant factors of the length of the interval, as the fully adversarial case. In other words, any small amount of imprecision in the ground truth dramatically complicates making calibrated predictions.
​
11:20 - 11:55: Talk 10 (Christoph Jansen)
Title: Statistical Multicriteria Benchmarking via the GSD-Front
Abstract: Given the vast number of classifiers that have been (and continue to be) proposed, reliable methods for comparing them are becoming increasingly important. The desire for reliability is broken down into three main aspects: (1) Comparisons should allow for different quality metrics simultaneously. (2) Comparisons should take into account the statistical uncertainty induced by the choice of benchmark suite. (3) The robustness of the comparisons under small deviations in the underlying assumptions should be verifiable. To address (1), we propose to compare classifiers using a generalized stochastic dominance ordering (GSD) and present the GSD-front as an information-efficient alternative to the classical Pareto-front. For (2), we propose a consistent statistical estimator for the GSD-front and construct a statistical test for whether a (potentially new) classifier lies in the GSD-front of a set of state-of-the-art classifiers. For (3), we relax our proposed test using techniques from robust statistics and imprecise probabilities. We illustrate our concepts on the benchmark suite PMLB and on the platform OpenML.
​
—---------------------------- Lunch Break —-----------------------------------------
14:00 - 14:35: Talk 11 (Julian Rodemann)
Title: Can Reciprocal Learning Converge?
Abstract: We demonstrate that a wide range of established machine learning algorithms are specific instances of one single paradigm: Reciprocal learning. These instances range from active learning over multi-armed bandits to self-training in semi-supervised learning. All these methods iteratively enhance training data in a way that depends on the current model fit. We study under what conditions reciprocal learning converges to a non-changing model. The key is to guarantee that reciprocal learning contracts such that the Banach fixed-point theorem holds. In this way, we find that reciprocal learning algorithms converge under (mild) assumptions on the loss function if and only if the data enhancement is either randomized or regularized -- as opposed to classical regularization of model fitting.
​
14:40 - 15:15: Talk 12 (Laura Iacovissi)
Title: Corruptions of Supervised Learning Problems
Abstract: Corruption is notoriously widespread in data collection. Despite extensive research, the existing literature on corruption predominantly focuses on specific settings and learning scenarios, lacking a unified view. To address that, we develop a taxonomy of corruption from an information-theoretic perspective – with Markov kernels as a foundational mathematical tool. We generalize the concept of corruption beyond dataset shift: corruption includes all modifications of a learning problem, while here we focus on changes in probability. We use the taxonomy to study the consequences of corruption on supervised learning tasks, by comparing Bayes risks in the clean and corrupted scenarios. Notably, while label corruptions affect only the loss function, more intricate cases involving attribute corruptions extend the influence beyond the loss to affect the hypothesis class. If time allows, I will also talk about how we leverage these insights to understand mitigations for various corruption types, in the form of loss correction techniques. This is joint work with Nan Lu and Bob Williamson.
​
15:10 - 15:45: Talk 13 (Armando Cabrera)
Title: Distorting losses, distorting sums
Abstract: The theory of aggregation functions has been widely studied and now we have at hand plenty of interesting and powerful results. This leads to the very natural question: why should we use the sum to aggregate losses in online learning? We study this question in the case of learning under expert advice. More precisely, we show that it is possible to adapt Vovk’s Aggregating Algorithm (AA) to fairly general aggregation functions. It turns out, these aggregations can be characterized as quasi-sums which can be interpreted as the learner’s attitude towards losses, e.g., risk aversion or risk seeking behavior. This suggests that a distortion of a loss function corresponds to a distortion of how we aggregate losses in the AA. This is joint work with R. Derr and R. Willliamson.
​
—---------------------------- Coffee Break —-----------------------------------------
​
​
16:15 - 16:50: Talk 14 (Benedikt Höltgen)
Title: Machine Learning Without True Probabilities
Abstract: Drawing on scholarship across disciplines, we argue that probabilities are constructed rather than discovered, that there are no true probabilities. Since existence of true probabilities is often implicitly or explicitly assumed in Machine Learning, this has implications for both theory and practice. True probability distributions may still be a useful theoretical framework for many settings but we argue that there are settings where alternative frameworks are needed. We show that urn models allow to prove Vapnik-style learning theory results without true probability distributions. We also strengthen the case that (probabilistic) Machine Learning models cannot be separated from the choices that went into their construction and from the task they were meant for.
​
16:55 - 17:30: Talk 15 (Hannah Blocher)
Title: Depth Functions for Non-Standard Data using Formal Concept Analysis
Abstract: Data depth functions have been studied extensively for normed vector spaces. However, a discussion of depth functions on data for which no specific data structure can be assumed is lacking. We call such data non-standard data. In order to define depth functions for non-standard data, we represent the data via formal concept analysis, which leads to a unified data representation. We provide a systematic basis for depth functions for non-standard data using formal concept analysis by introducing structural properties. We also introduce the union-free generic depth function, which is a generalisation of the simplicial depth function. This increases the number of spaces in which centrality is discussed. In particular, it provides a basis for defining further depth functions and statistical inference methods for non-standard data.
​
17:35 - 18:10: Talk 16 (Dominik Dürrschnabel)
Title: Order Dimension and Data Analysis
Abstract: Sets that are partially ordered, i.e., that entail not only comparability, but also incomparability, are a widely used mathematical model to describe dependencies in data. A measure for their complexity is the order dimension introduced by Dushnik and Miller. This dimension counts the minimum number of linear extensions that are needed to compose the order in their intersection. The set of these linear extensions is called the realizer of the order. In this talk, we are going to discuss several applications of the order dimension concept for data analysis. We demonstrate how the realizer can be used for data visualization purposes and introduce the concept of relative order dimension, an extension of the Dushnik-Miller order dimension concept that aims to be better tailored to data analysis purposes.
​
18:15 - 18:40: Talk 17 (Johannes Hirth und Tobias Hille)
Title: Conceptual Structures of Topic Models
Abstract: Topic modelling is a very active research field with many contributions in text mining, feature engineering and text clustering. These model embed (large) text corpora into a latent (topic) space. There are numerous methods and techniques to achieve this, e.g., with factorization, probabilistic, deep neural networks or large language models. Similarly, there are many methods to visualize and interpret the topic space. Despite their number, most of them have in common that they explained by extracting a binary relation between the dimensions of the topic space and words of the text corpus. With our talk, we present a new method to conceptually analyze the structure of the topic space based on this relation. This approach is based on Formal Concept Analysis and derives hierarchical structures from (flat) topic models. We demonstrate how this method can be applied to arbitrary topic model methods and how to interpret them by means of conceptual structures. For this, we employ a new method that is based on the identification of frequently occurring ordinal patterns.
​
​